Executive Summary
AI applications in modern businesses are transforming industries and driving innovation in today’s business landscape. “The AI Advantage: Real-World Use Cases for Modern Businesses” serves as a comprehensive guide for business leaders, entrepreneurs, and professionals. This book aims to help readers understand AI’s practical applications and harness its potential for a competitive edge.
The introduction outlines the book’s purpose and goals, emphasizing AI’s significance in modern business. It provides an overview of key AI technologies, including machine learning, natural language processing, and computer vision.
Chapter 1 delves into AI fundamentals, defining its subfields, tracing its historical development, and comparing AI with human intelligence. Key AI concepts such as algorithms, data, and training are explained to provide a solid foundation.
Subsequent chapters explore AI’s applications in specific industries, offering real-world case studies and highlighting the benefits and challenges of AI implementation. These chapters cover:
- Healthcare: AI’s role in diagnostics, treatment planning, and patient care, with examples like IBM Watson and AI-driven medical imaging.
- Finance: AI’s use in fraud detection, risk management, and personalized financial services, featuring robo-advisors and AI-driven trading algorithms.
- Retail and E-commerce: AI’s impact on personalized marketing, inventory management, and customer service, with examples like recommendation engines and chatbots.
- Manufacturing: AI’s applications in predictive maintenance, quality control, and automation, including smart factories and AI-driven supply chain optimization.
- Telecommunications: AI’s role in network optimization, customer service, and predictive maintenance, with examples like AI-driven network management and virtual assistants.
- Education: AI’s use in personalized learning, administrative automation, and student engagement, featuring adaptive learning platforms and AI-driven tutoring systems.
- Energy and Utilities: AI’s applications in optimizing energy consumption, predictive maintenance, and grid management, including smart grids and AI-driven energy management systems.
- Media and Entertainment: AI’s impact on content recommendation, audience analysis, and production automation, with examples like AI-driven content creation and personalized streaming services.
- Government and Public Sector: AI’s role in public services, security, and resource allocation, featuring AI-driven public safety systems and smart city initiatives.
- Autonomous Vehicles: AI’s use in self-driving cars, drones, and other autonomous systems, with examples like Tesla’s Autopilot and Waymo’s self-driving technology.
- Cybersecurity: AI’s applications in threat detection, incident response, and vulnerability management, featuring AI-driven threat intelligence platforms and automated security operations.
- Agriculture: AI’s role in precision farming, crop monitoring, and livestock management, with examples like AI-driven irrigation systems and crop yield prediction models.
- Human Resources: AI’s use in talent acquisition, employee engagement, and performance management, featuring AI-driven recruitment platforms and employee sentiment analysis tools.
- Legal Services: AI’s applications in legal research, contract analysis, and case prediction, with examples like AI-driven legal research platforms and automated contract review systems.
A dedicated chapter on AI ethics and governance addresses the ethical implications of AI, including data privacy, bias, and fairness. It also explores frameworks for responsible AI development and deployment.
The book concludes with practical guidance on implementing AI in businesses. It covers identifying AI opportunities, building an AI strategy, addressing ethical considerations, and exploring emerging trends. The final chapter recaps key points, discusses future trends in AI, and encourages readers to explore AI applications in their own industries.
With a glossary of AI terms, resources for further reading, and acknowledgments, “The AI Advantage” equips readers with the knowledge and insights needed to navigate the AI-driven future of business.
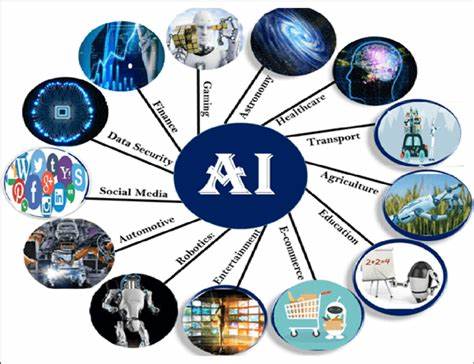
Introduction
AI applications in modern businesses are transforming industries and driving innovation. Welcome to “The AI Advantage: Real-World Use Cases for Modern Businesses.” This book will help you understand how artificial intelligence (AI) can transform your business. We’ll explore different AI technologies and show you how they are used in various industries.
Purpose of the Book
The goal of this book is to explain how AI can benefit your business. You’ll learn about different AI applications and see real-world examples of how companies use AI to improve their operations.
Why AI Matters
AI is changing the way businesses operate. It helps companies make better decisions, improve customer service, and increase efficiency. Understanding AI is essential for staying competitive in today’s business world.
Overview of AI Technologies
AI includes several key technologies:
- Machine Learning: This technology allows computers to learn from data and make predictions or decisions without being explicitly programmed.
- Natural Language Processing (NLP): NLP enables computers to understand and respond to human language.
- Computer Vision: This technology allows computers to interpret and understand visual information from the world, such as images and videos.
In the following chapters, we’ll dive deeper into these technologies and explore how they are used in different industries. You’ll see how AI is transforming healthcare, finance, retail, manufacturing, telecommunications, education, energy, media, government, autonomous vehicles, cybersecurity, agriculture, human resources, and legal services.
By the end of this book, you’ll have a solid understanding of AI and its potential to revolutionize your business. Let’s get started on this exciting journey into the world of AI!
Understanding AI
What is AI?
Artificial Intelligence (AI) is a branch of computer science that aims to create machines capable of performing tasks that usually require human intelligence. These tasks include learning, reasoning, problem-solving, and understanding language. AI can be divided into several subfields, such as machine learning, natural language processing, and computer vision.
History of AI
The history of AI dates back to the 1950s when scientists first began exploring the idea of creating intelligent machines. Early AI research focused on simple tasks like playing chess and solving mathematical problems. Over the years, AI has evolved significantly, thanks to advancements in computing power and data availability. Today, AI is used in various industries to solve complex problems and improve efficiency.
AI vs. Human Intelligence
AI and human intelligence have some similarities, but they also have key differences. Both can learn from experience and make decisions based on data. However, AI lacks emotions and consciousness, which are essential aspects of human intelligence. While AI can process vast amounts of data quickly, humans excel at understanding context and making intuitive decisions.
Key AI Concepts
To understand AI better, it’s important to know some key concepts:
- Algorithms: These are step-by-step instructions that tell a computer how to perform a task. In AI, algorithms help machines learn from data and make predictions or decisions.
- Data: AI systems rely on large amounts of data to learn and improve. This data can come from various sources, such as sensors, images, and text.
- Training: Training is the process of teaching an AI system to perform a specific task. During training, the system learns from data and adjusts its algorithms to improve its performance.
In the next chapters, we’ll explore how AI is used in different industries and see real-world examples of its applications. By understanding these basics, you’ll be better prepared to see how AI can transform your business.
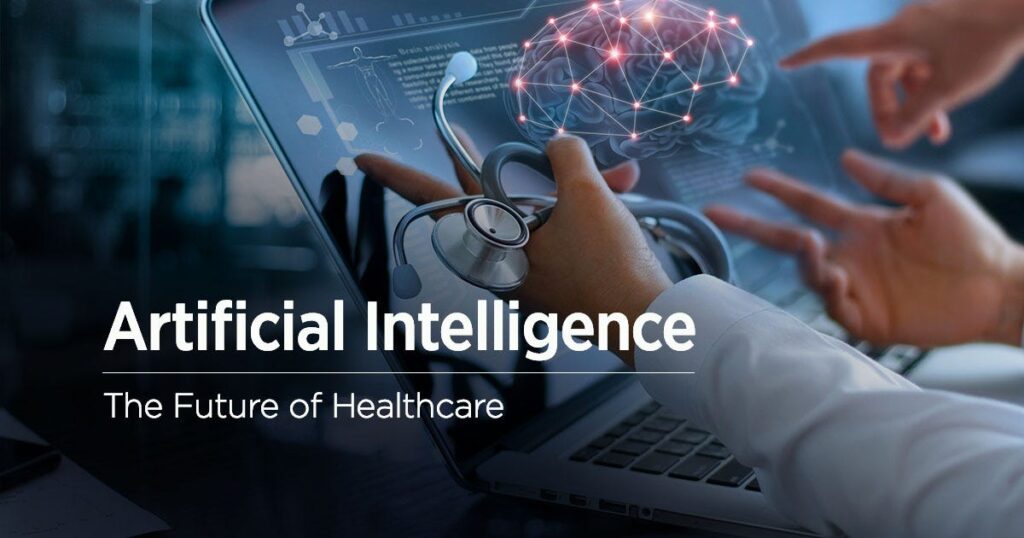
AI Applications for Specific Industries
AI Applications for Healthcare
Artificial Intelligence (AI) is making a big impact in healthcare. Doctors and hospitals use AI to improve patient care and make better decisions. Here are some ways AI is used in healthcare:
- Diagnostics: AI helps doctors diagnose diseases more accurately by analyzing medical images like X-rays, MRIs, and CT scans. This improves early detection and treatment of conditions such as cancer and cardiovascular diseases2.
- Predictive Analytics: AI predicts disease outbreaks and patient outcomes by analyzing large datasets. This helps healthcare providers prepare for and manage potential health crises3.
- Personalized Medicine: AI tailors treatments to individual patients based on their genetic makeup and other factors. This ensures that patients receive the most effective treatments for their specific conditions3.
- Virtual Health Assistants: AI-powered chatbots and virtual assistants provide 24/7 support to patients, answering questions and offering medical advice. This improves patient engagement and access to healthcare services2.
- Drug Discovery: AI accelerates the process of discovering new drugs by analyzing chemical compounds and predicting their effectiveness. This helps pharmaceutical companies develop new treatments more quickly and efficiently3.
These applications show how AI is revolutionizing healthcare, making it more efficient, accurate, and personalized.
Case Studies
Let’s look at some real-world examples of AI in healthcare:
Case Study 1: AI-Powered Diagnostics at Massachusetts General Hospital
Challenge: Detecting lung nodules in X-rays and CT scans with high accuracy was a significant challenge for radiologists at Massachusetts General Hospital (MGH).
Solution: MGH partnered with MIT to develop an AI system that analyzes medical images to detect lung nodules. Trained on thousands of images, the AI software recognizes patterns indicating lung nodules.
Result: Achieving a 94% accuracy rate in detecting lung nodules, the AI app significantly outperformed the 65% accuracy rate of human radiologists.
Case Study 2: AI in Eye Care at Moorfields Eye Hospital
Challenge: Moorfields Eye Hospital needed to analyze over 5,000 optical coherence tomography (OCT) scans per week to diagnose severe eye conditions like diabetic retinopathy and age-related macular degeneration (AMD).
Solution: Moorfields partnered with DeepMind to develop an AI tool capable of identifying more than 50 eye diseases. Trained on almost 15,000 OCT scans from 7,500 patients, the AI tool was designed to recognize a wide range of eye conditions.
Result: Achieving a 94% accuracy rate in diagnosing eye diseases, the AI tool matched the performance of top eye professionals.
Case Study 3: Early Detection of Sepsis at HCA Healthcare
Challenge: Detecting sepsis early is crucial for patient survival, but traditional methods were often too slow.
Solution: HCA Healthcare implemented an AI system that monitors patients’ vital signs and analyzes data from electronic health records to detect early signs of sepsis.
Result: The AI application provided early warnings of sepsis, allowing doctors to treat patients sooner and improving their chances of recovery.
Case Study 4: AI in Cancer Treatment at Memorial Sloan Kettering
Challenge: Creating personalized treatment plans for cancer patients was time-consuming and complex.
Solution: Memorial Sloan Kettering Cancer Center used IBM Watson for Oncology, an AI application that analyzes medical records, research papers, and clinical trial data to suggest the best treatment options. By leveraging this AI system, doctors could access a wealth of information quickly and efficiently.
Result: The implementation of IBM Watson for Oncology enabled doctors to create more informed and personalized treatment plans, significantly improving patient care.
Case Study 5: Predictive Analytics in Diabetes Management at UCSF
Challenge: Managing diabetes effectively requires constant monitoring of blood sugar levels, which can be challenging for patients.
Solution: The University of California, San Francisco (UCSF) developed an AI system that analyzes data from patients’ glucose monitors and predicts blood sugar levels. By providing accurate predictions, the system helps patients manage their diabetes more effectively and avoid complications.
Result: Accurate predictions from the AI app enabled patients to manage their diabetes more effectively, reducing the risk of complications.
Benefits and Challenges
AI offers many benefits in healthcare, but there are also some challenges to consider:
Benefits:
- Improved Accuracy: AI can analyze large amounts of data quickly and accurately, helping doctors make better decisions.
- Personalized Care: AI can create personalized treatment plans based on a patient’s unique medical history and condition.
- Efficiency: AI can automate routine tasks, freeing up doctors and nurses to focus on patient care.
Challenges:
- Data Privacy: Protecting patients’ personal information is crucial. AI systems must be designed to keep data secure.
- Bias: AI apps can sometimes be biased if they are trained on incomplete or unrepresentative data. It’s important to ensure that AI applications are fair and unbiased.
- Cost: Implementing AI in healthcare can be expensive. Hospitals and clinics need to invest in the right technology and training for their staff.
AI is transforming healthcare by improving diagnostics, treatment planning, and patient care. The case studies of Massachusetts General Hospital, Moorfields Eye Hospital, and HCA Healthcare demonstrate significant advancements in accuracy, early detection, and personalized treatment. While challenges like data privacy and implementation costs exist, the benefits of AI in healthcare make it essential for enhancing patient outcomes and efficiency.
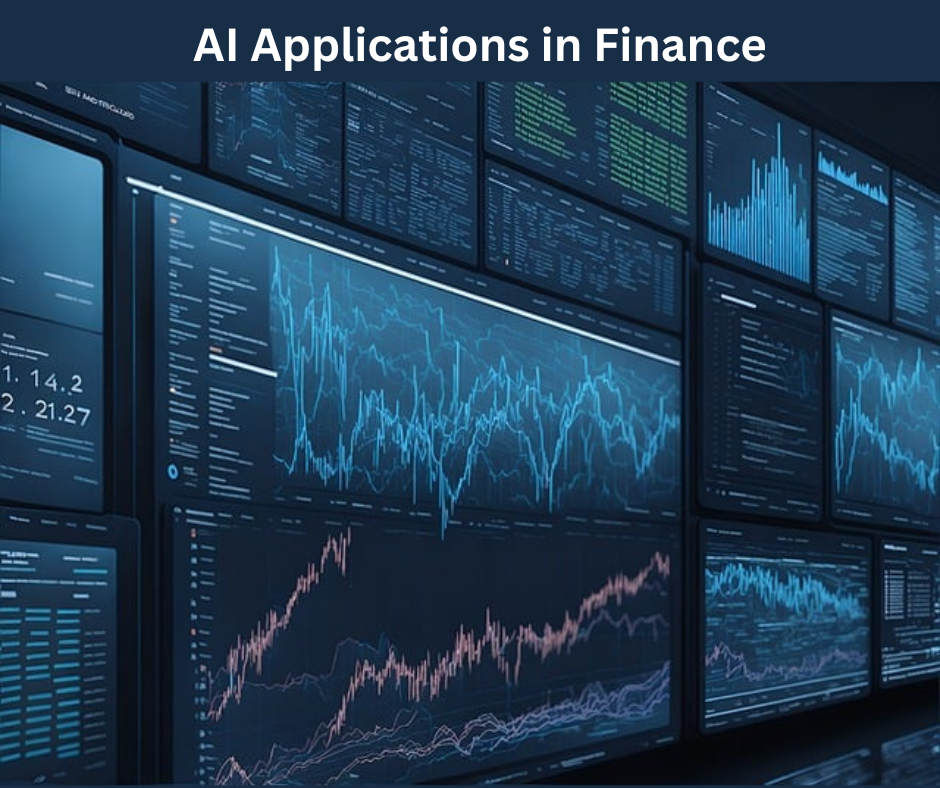
AI Applications for Finance
AI Applications in Finance
Artificial Intelligence (AI) is changing the finance industry in many ways. Banks and financial institutions use AI to improve their services and protect their customers. Here are some ways AI is used in finance:
Fraud Detection and Prevention: AI systems analyze vast amounts of transaction data in real-time to identify patterns and anomalies that may indicate fraudulent activity. This helps financial institutions protect their customers and reduce losses.
Credit Scoring and Risk Assessment: AI models assess credit risk more accurately by considering a wider range of data points, including unconventional data sources like utility payments and social media activity. This leads to better decision-making and reduced default rates.
Algorithmic Trading: AI-driven trading algorithms analyze market data and execute trades based on predefined strategies. This allows for faster and more accurate trading decisions, optimizing investment performance.
Customer Service Automation: AI-powered chatbots and virtual assistants provide 24/7 customer support, addressing queries and resolving issues promptly. This improves customer satisfaction and reduces operational costs.
Personalized Financial Services: AI offers personalized financial advice and services based on individual customer data. This includes robo-advisors that help manage investment portfolios and provide tailored recommendations.
These applications demonstrate how AI is transforming the finance industry, making it more efficient, secure, and customer centric.
Case Studies
Let’s look at some real-world examples of AI in finance:
Case Study 1: AI-Driven Fraud Detection at FinSecure Bank
Challenge: FinSecure Bank faced significant challenges with financial fraud, leading to substantial annual losses and diminished customer trust. Traditional rule-based systems were inefficient, with high false positive rates and an inability to adapt to evolving fraudulent tactics.
Solution: FinSecure Bank implemented an advanced AI-driven solution using machine learning models to enhance its fraud detection capabilities. By analyzing vast amounts of real-time transaction data, the system identified patterns and anomalies that indicated potential fraud. It used a combination of supervised and unsupervised learning techniques to recognize known fraud patterns and identify new, unusual behaviors.
Result: The implementation of the AI-driven fraud detection system resulted in a 60% reduction in fraudulent activities within the first year.
Case Study 2: AI-Driven Credit Risk Assessment at FinScore Global
Challenge: FinScore Global, a financial services firm, faced challenges in accurately assessing credit risk for a diverse global client base. Traditional models often failed to account for unique financial behaviors and risk factors across different geographic and demographic segments.
Solution: FinScore Global adopted a generative AI model designed to simulate various credit scenarios based on extensive data inputs, including unconventional data sources like utility payments and social media activity. The AI system generated predictive credit scoring models that adapted to regional financial behaviors and trends, allowing for more nuanced risk assessments.
Result: The implementation of generative AI in credit risk assessment led to a 25% reduction in default rates and a 40% increase in credit issuance to previously underserved segments.
Case Study 3: AI Optimization in Investment Strategies at Quantum Capital
Challenge: Quantum Capital, an investment management firm, sought to optimize its investment strategies to maximize returns and minimize risks. Traditional methods were time-consuming and often failed to account for rapidly changing market conditions.
Solution: Quantum Capital incorporated AI and machine learning into its investment strategies. The AI system analyzed vast amounts of market data, identified trends, and executed trades based on predefined strategies. This allowed the firm to make faster and more accurate trading decisions.
Result: The AI-driven investment strategies led to significant improvements in portfolio performance, with higher returns and reduced risks.
Benefits and Challenges
AI offers many benefits in finance, but there are also some challenges to consider:
Benefits:
- Improved Security: AI can detect and prevent fraud more effectively than traditional methods, protecting customers and financial institutions.
- Better Decision-Making: AI can analyze large amounts of data quickly, helping financial institutions make better decisions and manage risks more effectively.
- Personalized Services: AI can provide personalized financial advice and services, helping customers achieve their financial goals.
Challenges:
- Data Privacy: Protecting customers’ personal information is crucial. AI systems must be designed to keep data secure.
- Bias: AI applications can sometimes be biased if they are trained on incomplete or unrepresentative data. It’s important to ensure that AI systems are fair and unbiased.
- Regulation: The finance industry is heavily regulated, and AI apps must comply with these regulations. This can be challenging and time-consuming.
AI is transforming the finance industry by enhancing fraud detection, improving customer service, and optimizing investment strategies. The case studies of FinSecure Bank, FinScore Global, and Quantum Capital demonstrate significant improvements in security, credit risk assessment, and investment performance. While challenges like data privacy and regulatory compliance exist, the benefits of AI in finance make it essential for staying competitive.
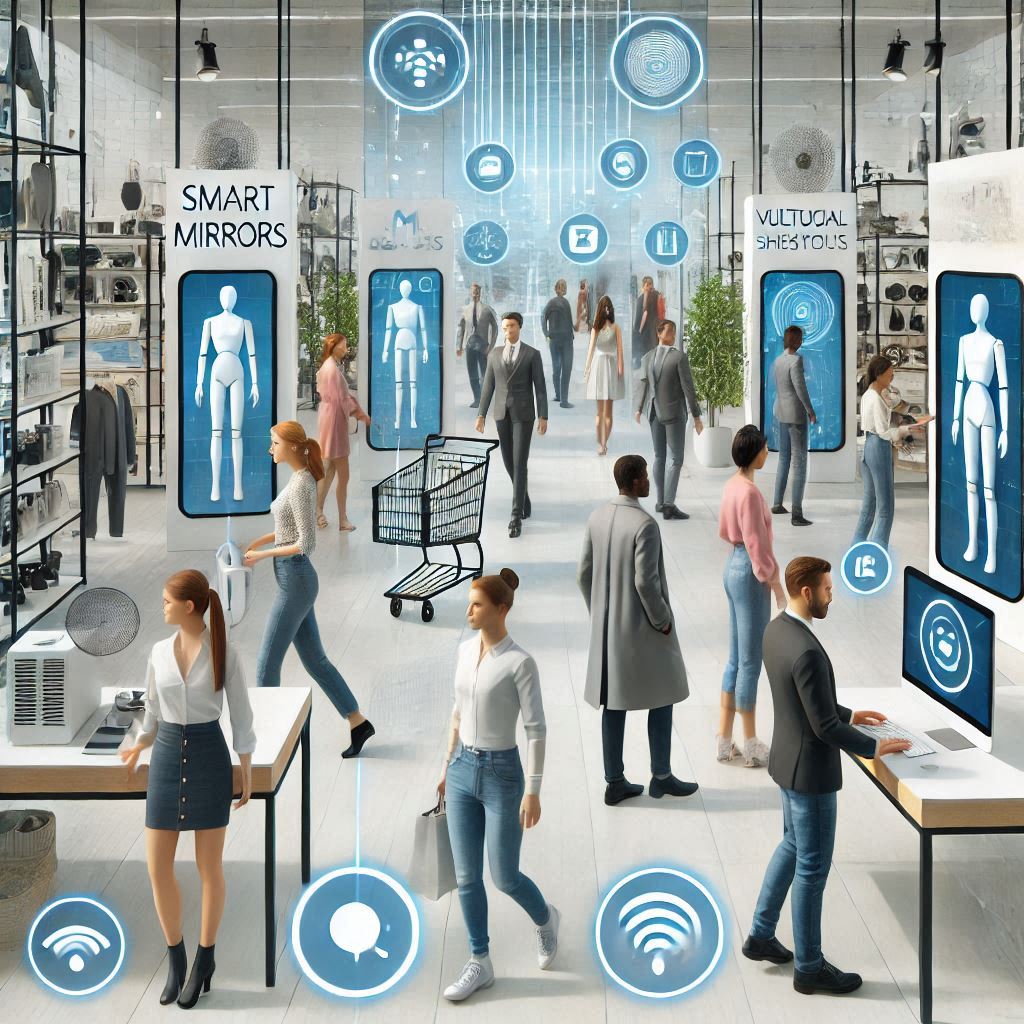
AI Applications for Retail and E-commerce
AI Applications in Retail
Artificial Intelligence (AI) is transforming the retail and e-commerce industry. Businesses use AI to improve customer experiences, manage inventory, and boost sales. Here are some ways AI is used in retail:
- Personalized Marketing: AI analyzes customer data to create personalized marketing campaigns, targeting the right customers with the right products.
- Inventory Management: AI predicts demand and helps manage inventory levels, ensuring popular products are always in stock and reducing waste.
- Customer Service: AI-powered chatbots and virtual assistants provide 24/7 customer support, answering questions, processing orders, and resolving issues quickly.
- Demand Forecasting: AI predicts future buying trends by analyzing customer purchase data and supply chain analytics.
- Price Optimization: AI adjusts prices in real-time based on factors like demand, competition, and inventory levels to maximize sales and profits.
- Product Recommendations: AI suggests products to customers based on their browsing and purchase history, increasing sales and customer satisfaction.
Case Studies
Case Study 1: Personalized Marketing at ShopSmart
Challenge: ShopSmart, an online retailer, struggled to engage customers with generic marketing campaigns. They needed a way to personalize their marketing efforts to increase sales.
Solution: ShopSmart implemented an AI system that analyzes customer data, such as browsing history and purchase behavior. The AI system creates personalized marketing campaigns tailored to each customer’s preferences.
Result: The personalized marketing campaigns led to a 30% increase in sales and a 20% improvement in customer engagement.
Case Study 2: Inventory Management at FreshMart
Challenge: FreshMart, a grocery store chain, faced challenges with managing inventory levels. They often ran out of popular products or had excess stock of less popular items.
Solution: FreshMart adopted an AI system that predicts demand based on historical sales data and external factors like weather and holidays. The AI system helps FreshMart manage inventory levels more effectively.
Result: The AI-driven inventory management system reduced stockouts by 40% and decreased excess inventory by 25%.
Case Study 3: Customer Service at TechGear
Challenge: TechGear, an electronics retailer, struggled to provide timely customer support. Long wait times and unresolved issues led to customer dissatisfaction.
Solution: TechGear implemented an AI-powered chatbot that provides 24/7 customer support. The chatbot can answer common questions, process orders, and resolve issues quickly.
Result: The AI-powered chatbot reduced customer wait times by 50% and improved customer satisfaction by 35%.
Benefits and Challenges
AI offers many benefits in retail, but there are also some challenges to consider:
Benefits:
- Improved Customer Experience: AI can personalize marketing and provide better customer support, leading to happier customers.
- Efficient Inventory Management: AI helps businesses manage inventory levels more effectively, reducing stockouts and excess inventory.
- Increased Sales: AI-driven marketing campaigns can boost sales by targeting the right customers with the right products.
Challenges:
- Data Privacy: Protecting customer data is crucial. AI systems must be designed to keep data secure.
- Bias: AI applications can sometimes be biased if they are trained on incomplete or unrepresentative data. It’s important to ensure that AI apps are fair and unbiased.
- Cost: Implementing AI in retail can be expensive. Businesses need to invest in the right technology and training for their staff.
AI is transforming retail and e-commerce by enhancing personalized marketing, improving inventory management, and boosting customer service. The case studies of ShopSmart, FreshMart, and TechGear demonstrate significant improvements in sales, inventory efficiency, and customer satisfaction. While challenges like data privacy and implementation costs exist, the benefits of AI in retail make it essential for staying competitive.
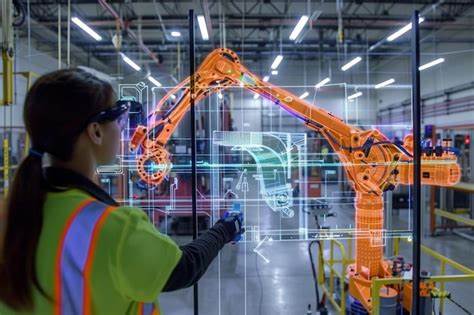
AI Applications for Manufacturing
Artificial Intelligence (AI) is revolutionizing the manufacturing industry. By leveraging AI, manufacturers can enhance efficiency, reduce costs, and improve product quality. This chapter explores the various applications of AI in manufacturing, highlighting real-world case studies and discussing the benefits and challenges of AI implementation.
AI Applications in Manufacturing
AI is transforming manufacturing processes in several ways. Here are some of the top AI applications in manufacturing:
Predictive Maintenance: AI algorithms analyze data from machinery to predict when maintenance is needed. This helps prevent unexpected breakdowns and reduces downtime2.
Quality Control: AI-powered systems inspect products for defects with high accuracy. This ensures consistent product quality and reduces waste2.
Supply Chain Optimization: AI optimizes supply chain processes by predicting demand, managing inventory, and optimizing routes. This improves efficiency and reduces costs.
Generative Design: AI uses machine learning algorithms to generate design options based on specified parameters. This allows manufacturers to quickly explore multiple design possibilities and innovate faster.
Robotics and Automation: AI-driven robots and machines perform repetitive tasks with precision. This increases production speed, reduces human error, and allows human workers to focus on more complex tasks2.
Case Studies
Case Study 1: General Electric (GE)
- Challenge: GE faced frequent equipment failures, leading to costly downtime and maintenance expenses.
- Solution: GE implemented AI-powered predictive maintenance systems. These systems analyzed data from sensors on equipment to predict failures before they occurred.
- Result: GE reduced unplanned downtime by 20% and maintenance costs by 10%.
Case Study 2: BMW
- Challenge: BMW needed to ensure high-quality standards in its manufacturing process while reducing inspection time.
- Solution: BMW deployed AI-powered quality control systems. These systems used computer vision to inspect parts and identify defects in real-time.
- Result: BMW improved inspection accuracy by 99% and reduced inspection time by 30%.
Case Study 3: Siemens
- Challenge: Siemens aimed to increase production efficiency and reduce operational costs in its factories.
- Solution: Siemens implemented AI-driven automation solutions. These solutions included AI-powered robots and machines that performed repetitive tasks with high precision.
- Result: Siemens increased production efficiency by 15% and reduced operational costs by 12%.
Benefits and Challenges
Benefits:
- Increased Efficiency: AI optimizes manufacturing processes, leading to higher efficiency and productivity.
- Cost Reduction: AI helps reduce operational costs by minimizing downtime and improving resource utilization.
- Improved Quality: AI-powered quality control ensures consistent product quality and reduces defects.
Challenges:
- Implementation Costs: The initial investment in AI technology can be high.
- Data Privacy: Ensuring the privacy and security of data used by AI systems is crucial.
- Skill Gap: There is a need for skilled personnel to develop, implement, and maintain AI applications.
AI is revolutionizing manufacturing by enhancing efficiency, reducing costs, and improving product quality. The case studies of General Electric, BMW, and Siemens demonstrate significant improvements in predictive maintenance, quality control, and automation. While challenges like implementation costs and data privacy exist, the benefits of AI in manufacturing make it essential for staying competitive.
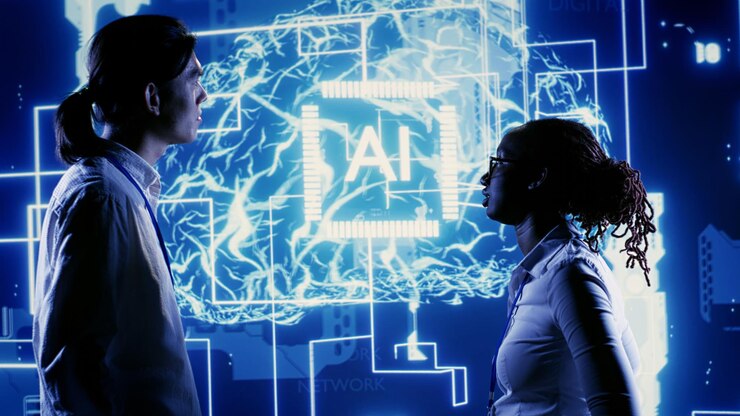
AI Applications for Telecommunications
Artificial Intelligence (AI) is transforming the telecommunications industry. By leveraging AI, telecom companies can optimize networks, enhance customer service, and predict maintenance needs. This chapter explores the various applications of AI in telecommunications, highlighting real-world case studies and discussing the benefits and challenges of AI implementation.
AI Applications in Telecommunications
AI is revolutionizing telecommunications in several ways. Here are some of the top AI applications in Telecommunications:
- Network Optimization: AI algorithms analyze network data to optimize performance and manage traffic. This ensures a seamless experience for users.
- Customer Service: AI-powered chatbots and virtual assistants provide 24/7 customer support. They handle common queries, process requests, and resolve issues quickly.
- Predictive Maintenance: AI predicts equipment failures by analyzing data from network components. This helps prevent outages and reduces maintenance costs.
- Fraud Detection: AI systems detect fraudulent activities by analyzing patterns and anomalies in data. This helps telecom companies protect their networks and customers.
- Personalized Marketing: AI analyzes customer data to create personalized marketing campaigns. This targets the right customers with the right products, improving engagement and sales.
These applications demonstrate how AI is revolutionizing telecommunications by optimizing networks, enhancing customer service, and improving overall efficiency.
Case Studies
Case Study 1: AT&T
- Challenge: AT&T faced network congestion and performance issues, leading to customer dissatisfaction.
- Solution: AT&T implemented AI-driven network optimization tools. These tools analyzed network data in real-time to manage traffic and optimize performance.
- Result: AT&T improved network performance by 30% and reduced customer complaints by 25%.
Case Study 2: Vodafone
- Challenge: Vodafone needed to enhance customer service and reduce response times.
- Solution: Vodafone deployed AI-powered chatbots to handle customer queries and support requests. The chatbots provided instant responses and resolved common issues.
- Result: Vodafone reduced customer service response times by 50% and improved customer satisfaction by 35%.
Case Study 3: Verizon
- Challenge: Verizon aimed to reduce equipment failures and maintenance costs.
- Solution: Verizon implemented AI-based predictive maintenance systems. These systems analyzed data from network components to predict failures and schedule maintenance.
- Result: Verizon reduced equipment failures by 20% and maintenance costs by 15%.
Benefits and Challenges
Benefits:
- Improved Network Performance: AI optimizes network performance, ensuring a seamless user experience.
- Enhanced Customer Service: AI-powered chatbots provide quick and efficient customer support.
- Cost Reduction: AI helps reduce maintenance costs by predicting equipment failures and scheduling maintenance.
Challenges:
- Implementation Costs: The initial investment in AI technology can be high.
- Data Privacy: Ensuring the privacy and security of data used by AI systems is crucial.
- Skill Gap: There is a need for skilled personnel to develop, implement, and maintain AI applications.
AI is revolutionizing telecommunications by optimizing networks, enhancing customer service, and predicting maintenance needs. The case studies of AT&T, Vodafone, and Verizon show significant improvements in performance and cost reduction. While challenges like implementation costs and data privacy exist, the benefits of AI in telecommunications make it essential for staying competitive.
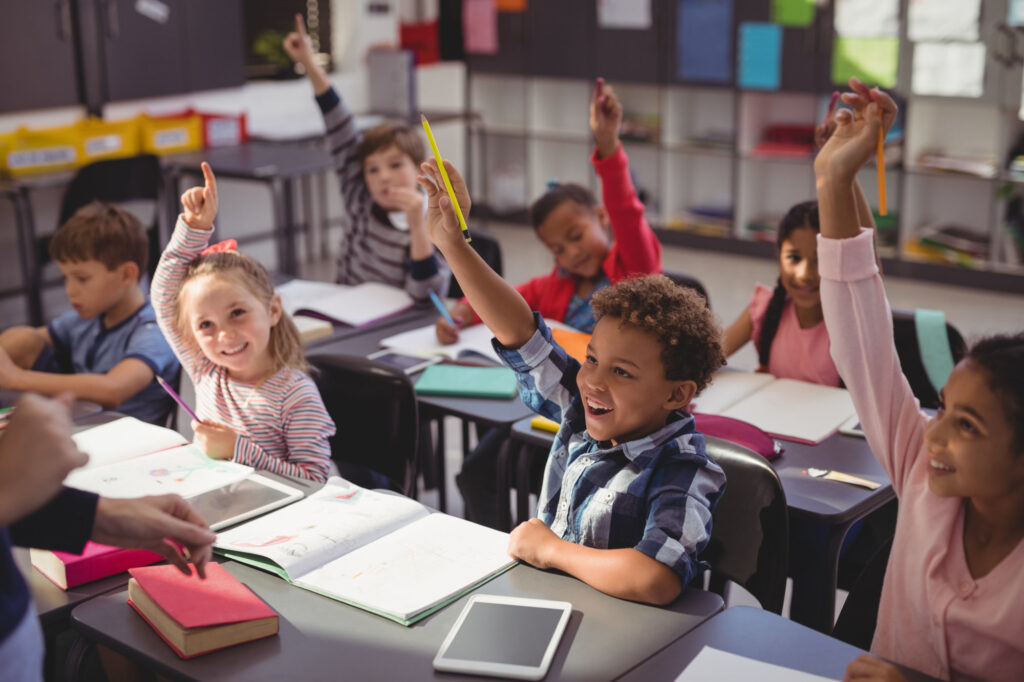
AI Applications for Education
Artificial Intelligence (AI) is revolutionizing the education sector. By leveraging AI, educators can personalize learning, automate administrative tasks, and enhance student engagement. This chapter explores the various applications of AI in education, highlighting real-world case studies and discussing the benefits and challenges of AI implementation.
AI Applications in Education
AI is transforming education in several impactful ways. Personalized learning, administrative automation, and student engagement are key areas where AI is making a significant difference.
Personalized Learning: AI tailors educational content to each student’s unique learning style and pace. Platforms like DreamBox and Smart Sparrow adapt lessons dynamically, enabling students to master concepts at their own speed.
Automated Administrative Tasks: AI automates grading, scheduling, and report generation, significantly reducing the workload on educators. Tools like Gradescope provide consistent and objective grading of assignments.
Intelligent Tutoring Systems: AI-powered tutoring systems provide personalized instruction and feedback to students, helping them understand complex concepts and improve their performance.
Enhanced Accessibility: AI-driven assistive technologies support students with disabilities, ensuring a more inclusive learning environment. Speech recognition software transcribes spoken words into text for hearing-impaired students.
Data-Driven Insights: AI analyzes vast amounts of educational data to provide educators with actionable insights. Platforms like Knewton Alta track student performance across various metrics, helping teachers identify learning gaps and adjust their instructional strategies accordingly.
Case Studies
Case Study 1: Carnegie Learning
- Challenge: Carnegie Learning needed to improve math education outcomes for students.
- Solution: They implemented an AI-driven adaptive learning platform. This platform analyzed student performance and adjusted the difficulty level of math problems accordingly.
- Result: Students using the platform showed a 20% improvement in math scores compared to those using traditional methods.
Case Study 2: Georgia State University
- Challenge: Georgia State University aimed to reduce student dropout rates.
- Solution: The university deployed an AI-powered predictive analytics system. This system identified students at risk of dropping out and provided targeted support.
- Result: The university reduced dropout rates by 30% and increased graduation rates by 10%.
Case Study 3: Duolingo
- Challenge: Duolingo wanted to enhance language learning for its users.
- Solution: They integrated AI-driven tutoring systems into their platform. These systems provided personalized feedback and adaptive learning paths.
- Result: Users experienced a 25% increase in language proficiency and higher engagement levels.
Benefits and Challenges
Benefits:
- Enhanced Learning Outcomes: AI personalizes learning experiences, leading to better student performance.
- Increased Efficiency: AI automates administrative tasks, allowing educators to focus on teaching.
- Improved Engagement: AI-powered tools make learning more interactive and engaging.
Challenges:
- Implementation Costs: The initial investment in AI technology can be high.
- Data Privacy: Ensuring the privacy and security of student data is crucial.
- Skill Gap: There is a need for skilled personnel to develop, implement, and maintain AI systems.
By leveraging AI, the education sector can enhance learning outcomes, increase efficiency, and improve student engagement. Addressing the challenges and embracing the benefits will ensure a brighter future for education.
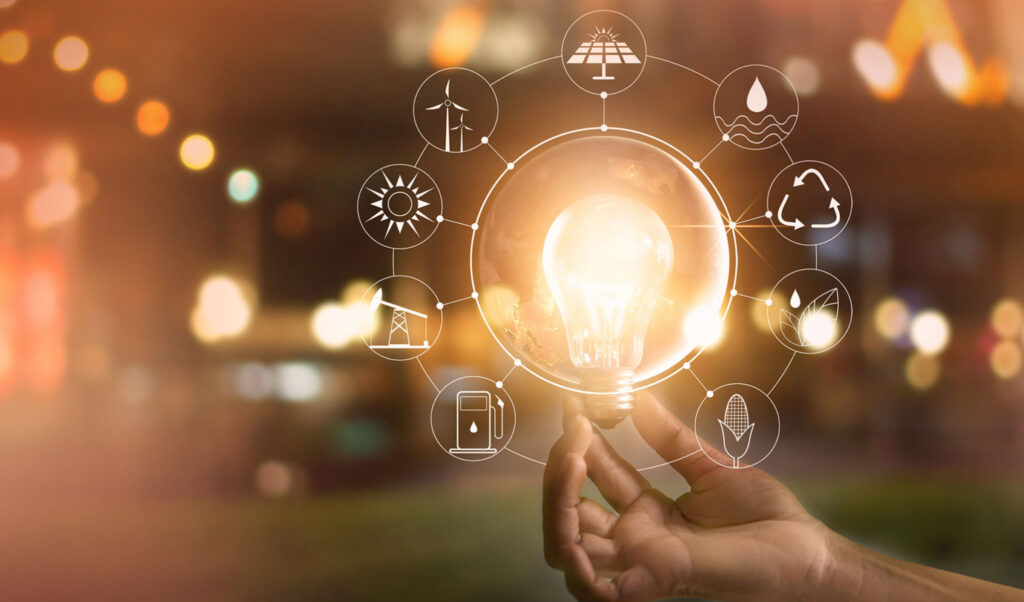
AI Applications for Energy and Utilities
Artificial Intelligence (AI) is revolutionizing the energy and utilities sector. By leveraging AI, companies can optimize energy consumption, predict maintenance needs, and manage grids more efficiently. This chapter explores the various applications of AI in energy and utilities, highlighting real-world case studies and discussing the benefits and challenges of AI implementation.
AI Applications in Energy and Utilities
AI is transforming the energy and utilities industry in several impactful ways. Here are the top 10 AI applications in this sector:
- Smart Grids: AI optimizes the distribution of electricity, balancing supply and demand in real-time.
- Predictive Maintenance: AI predicts equipment failures by analyzing data from sensors, reducing downtime and maintenance costs.
- Energy Consumption Optimization: AI analyzes usage patterns to optimize energy consumption, reducing costs and improving efficiency.
- Renewable Energy Management: AI manages renewable energy sources like solar and wind, predicting output and optimizing integration into the grid.
- Demand Response: AI adjusts energy usage during peak times to prevent overloads and reduce costs.
- Fault Detection: AI detects faults in the grid quickly, minimizing outages and improving reliability.
- Energy Trading: AI analyzes market data to optimize energy trading strategies, maximizing profits.
- Customer Service: AI-powered chatbots provide 24/7 customer support, addressing queries and resolving issues promptly.
- Load Forecasting: AI predicts future energy demand, helping utilities plan and manage resources more effectively.
- Grid Security: AI detects and responds to cyber threats, protecting the grid from attacks.
Case Studies
Let’s look at some real-world examples of AI in energy and utilities:
Case Study 1: Smart Grid Management at Southern California Edison
- Challenge: Southern California Edison (SCE) faced challenges in balancing electricity supply and demand, leading to inefficiencies and potential outages.
- Solution: SCE implemented an AI-driven smart grid management system. The AI system analyzed real-time data from sensors and adjusted the distribution of electricity to balance supply and demand.
- Result: The AI system improved grid efficiency by 20% and reduced the frequency of outages by 15%.
Case Study 2: Predictive Maintenance at Duke Energy
- Challenge: Duke Energy experienced frequent equipment failures, leading to costly downtime and maintenance expenses.
- Solution: Duke Energy adopted an AI-powered predictive maintenance system. The AI system analyzed data from sensors on equipment to predict failures before they occurred.
- Result: The AI system reduced unplanned downtime by 25% and maintenance costs by 18%.
Case Study 3: Renewable Energy Management at NextEra Energy
- Challenge: NextEra Energy needed to manage the integration of renewable energy sources like solar and wind into the grid.
- Solution: NextEra Energy implemented an AI system that predicted the output of renewable energy sources and optimized their integration into the grid.
- Result: The AI system increased the efficiency of renewable energy integration by 30% and reduced reliance on non-renewable energy sources by 10%.
Benefits and Challenges
AI offers many benefits in the energy and utilities sector, but there are also some challenges to consider:
Benefits:
- Increased Efficiency: AI optimizes energy consumption and grid management, leading to higher efficiency and lower costs.
- Improved Reliability: AI detects faults and predicts maintenance needs, reducing outages and improving reliability.
- Enhanced Customer Service: AI-powered chatbots provide quick and efficient customer support.
Challenges:
- Implementation Costs: The initial investment in AI technology can be high.
- Data Privacy: Ensuring the privacy and security of data used by AI systems is crucial.
- Skill Gap: There is a need for skilled personnel to develop, implement, and maintain AI applications.
AI is transforming the energy and utilities sector by optimizing energy consumption, predicting maintenance needs, and managing grids more efficiently. The case studies of Southern California Edison, Duke Energy, and NextEra Energy demonstrate significant improvements in efficiency, reliability, and renewable energy integration. While challenges like implementation costs and data privacy exist, the benefits of AI in energy and utilities make it essential for staying competitive.
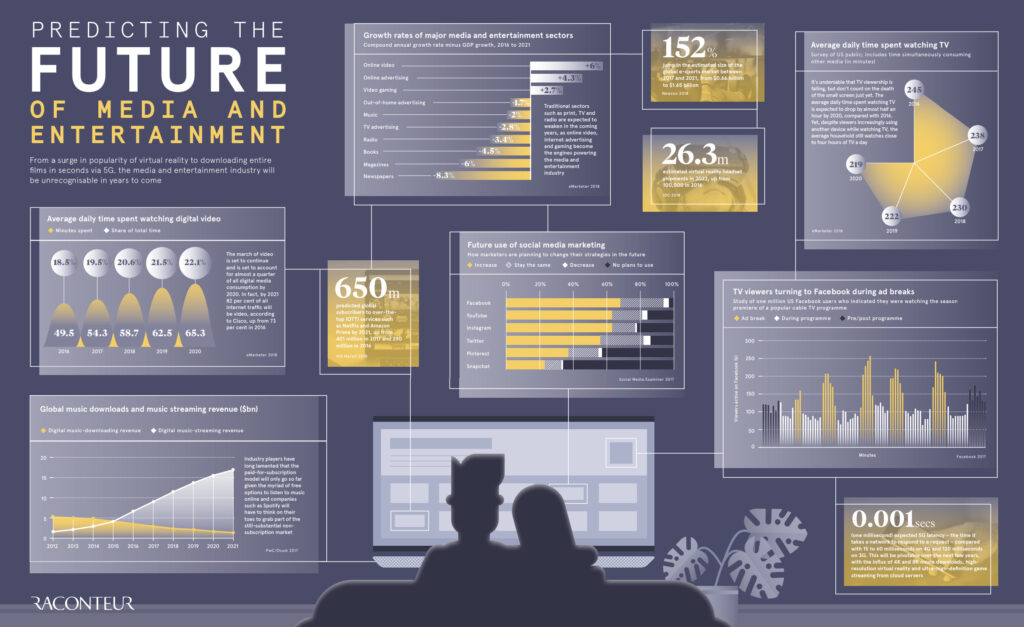
AI Applications for Media and Entertainment
Artificial Intelligence (AI) is revolutionizing the media and entertainment industry. By leveraging AI, companies can enhance content creation, improve audience engagement, and streamline production processes. This chapter explores the various applications of AI in media and entertainment, highlighting real-world case studies and discussing the benefits and challenges of AI implementation.
AI Applications in Media and Entertainment
AI is transforming media and entertainment in several impactful ways. Here are the top 10 AI applications in this sector:
- Content Recommendation: AI analyzes user preferences to recommend personalized content, improving user engagement.
- Audience Analysis: AI analyzes audience data to understand viewer behavior and preferences, helping companies tailor their content.
- Production Automation: AI automates various production tasks, such as editing and special effects, reducing time and costs.
- Content Creation: AI generates content, such as articles, music, and videos, enhancing creativity and productivity.
- Sentiment Analysis: AI analyzes social media and other platforms to gauge audience sentiment and feedback.
- Voice and Speech Recognition: AI enables voice-controlled interfaces and enhances accessibility for users.
- Ad Targeting: AI analyzes user data to deliver targeted advertisements, increasing ad effectiveness.
- Virtual Reality (VR) and Augmented Reality (AR): AI enhances VR and AR experiences, making them more immersive and interactive.
- Copyright Protection: AI detects and prevents copyright infringement, protecting intellectual property.
- Live Streaming: AI optimizes live streaming quality and provides real-time analytics to improve viewer experience.
Case Studies
Let’s look at some real-world examples of AI in media and entertainment:
Case Study 1: Content Recommendation at Netflix
- Challenge: Netflix needed to improve user engagement by recommending personalized content to its diverse audience.
- Solution: Netflix implemented an AI-driven recommendation system that analyzes user preferences and viewing history. The AI system suggests personalized content for each user.
- Result: The AI recommendation system increased user engagement by 20% and reduced churn rates by 15%.
Case Study 2: Production Automation at Warner Bros.
- Challenge: Warner Bros. faced challenges in managing the time-consuming and costly post-production process.
- Solution: Warner Bros. adopted an AI-powered production automation system. The AI system automated tasks such as editing, color correction, and special effects.
- Result: The AI system reduced post-production time by 30% and lowered costs by 25%.
Case Study 3: Sentiment Analysis at Disney
- Challenge: Disney needed to understand audience sentiment and feedback to improve its content and marketing strategies.
- Solution: Disney implemented an AI-driven sentiment analysis system. The AI system analyzed social media and other platforms to gauge audience sentiment.
- Result: The AI system provided valuable insights that helped Disney improve its content and marketing strategies, leading to a 15% increase in audience satisfaction.
Benefits and Challenges
AI offers many benefits in the media and entertainment sector, but there are also some challenges to consider:
Benefits:
- Enhanced Content Creation: AI enhances creativity and productivity by generating content and automating production tasks.
- Improved Audience Engagement: AI analyzes user preferences and behavior to deliver personalized content and targeted advertisements.
- Cost and Time Efficiency: AI automates various tasks, reducing production time and costs.
Challenges:
- Data Privacy: Ensuring the privacy and security of user data is crucial.
- Implementation Costs: The initial investment in AI technology can be high.
- Bias and Fairness: AI systems must be designed to be fair and unbiased, avoiding discrimination and ensuring inclusivity.
AI is transforming the media and entertainment sector by enhancing content creation, improving audience engagement, and streamlining production processes. The case studies of Netflix, Warner Bros., and Disney demonstrate significant improvements in user engagement, production efficiency, and audience satisfaction. While challenges like data privacy and implementation costs exist, the benefits of AI in media and entertainment make it essential for staying competitive.
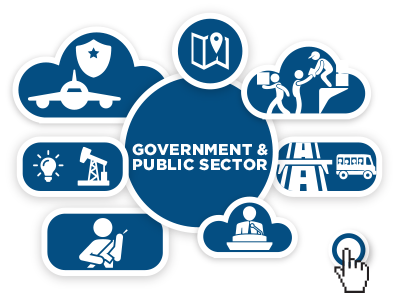
AI Applications for Gov and Public Sector
Artificial Intelligence (AI) is transforming the government and public sector. By leveraging AI, public institutions can improve services, enhance security, and optimize resource allocation. This chapter explores the various applications of AI in government and public sector, highlighting real-world case studies and discussing the benefits and challenges of AI implementation.
AI Applications in Government and Public Sector
AI is revolutionizing the government and public sector in several impactful ways. Here are the top 10 AI applications in this sector:
- Public Safety: AI analyzes data to predict and prevent crimes, enhancing public safety.
- Resource Allocation: AI optimizes the allocation of resources, ensuring efficient use of public funds.
- Traffic Management: AI monitors and manages traffic flow, reducing congestion and improving transportation efficiency.
- Citizen Services: AI-powered chatbots provide 24/7 support, answering citizen queries and processing requests.
- Disaster Response: AI predicts natural disasters and coordinates response efforts, minimizing damage and saving lives.
- Healthcare Services: AI improves public health by analyzing data to predict disease outbreaks and optimize healthcare delivery.
- Education: AI personalizes learning and automates administrative tasks, enhancing the education system.
- Environmental Monitoring: AI monitors environmental data to detect pollution and manage natural resources.
- Fraud Detection: AI detects fraudulent activities in public services, protecting public funds.
- Infrastructure Management: AI optimizes the maintenance and management of public infrastructure, reducing costs and improving efficiency.
Case Studies
Let’s look at some real-world examples of AI in government and public sector:
Case Study 1: Public Safety at New York Police Department (NYPD)
- Challenge: The NYPD faced challenges in predicting and preventing crimes, leading to increased crime rates.
- Solution: The NYPD implemented an AI-driven predictive policing system. The AI system analyzed crime data to identify patterns and predict potential crime hotspots.
- Result: The AI system helped reduce crime rates by 20% and improved the efficiency of police patrols.
Case Study 2: Disaster Response at Federal Emergency Management Agency (FEMA)
- Challenge: FEMA needed to improve its disaster response efforts to minimize damage and save lives.
- Solution: FEMA adopted an AI-powered disaster response system. The AI system analyzed data from various sources to predict natural disasters and coordinate response efforts.
- Result: The AI system improved disaster response times by 30% and reduced the impact of natural disasters.
Case Study 3: Traffic Management at Transport for London (TfL)
- Challenge: TfL faced challenges in managing traffic flow, leading to congestion and delays.
- Solution: TfL implemented an AI-driven traffic management system. The AI system monitored traffic data in real-time and optimized traffic signals to improve flow.
- Result: The AI system reduced traffic congestion by 25% and improved transportation efficiency.
Benefits and Challenges
AI offers many benefits in the government and public sector, but there are also some challenges to consider:
Benefits:
- Improved Public Services: AI enhances the delivery of public services, improving efficiency and citizen satisfaction.
- Enhanced Security: AI improves public safety by predicting and preventing crimes.
- Cost Efficiency: AI optimizes resource allocation and infrastructure management, reducing costs.
Challenges:
- Data Privacy: Ensuring the privacy and security of citizen data is crucial.
- Implementation Costs: The initial investment in AI technology can be high.
- Skill Gap: There is a need for skilled personnel to develop, implement, and maintain AI applications.
AI is transforming the government and public sector by improving services, enhancing security, and optimizing resource allocation. The case studies of the NYPD, FEMA, and TfL demonstrate significant improvements in public safety, disaster response, and traffic management. While challenges like data privacy and implementation costs exist, the benefits of AI in government and public sector make it essential for staying competitive.
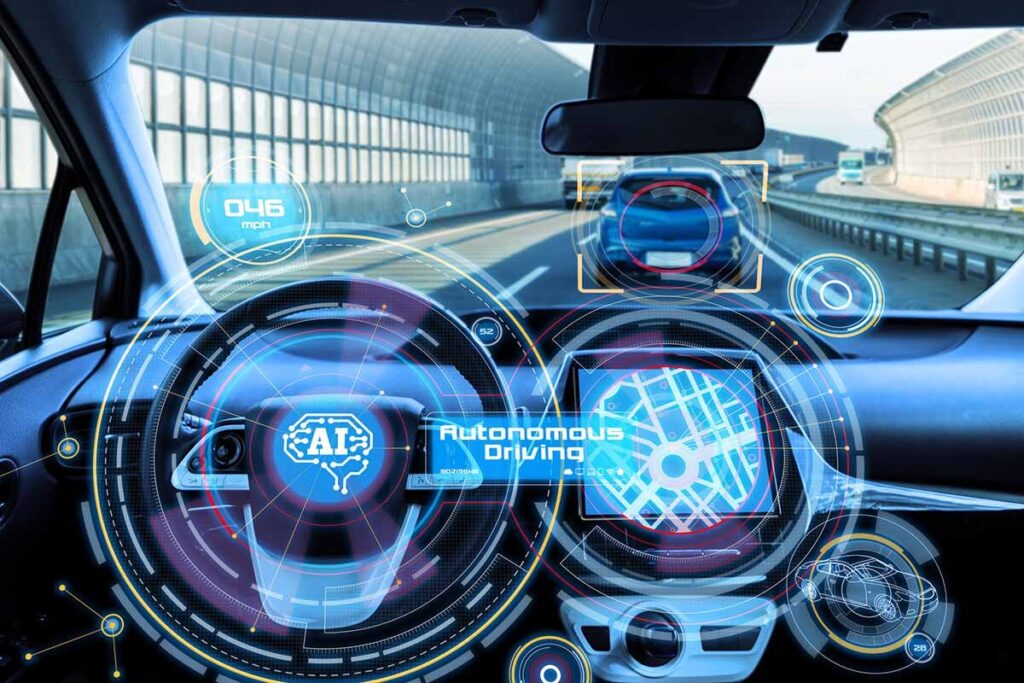
AI Applications for Autonomous Vehicles
Top 10 Applications of AI in Autonomous Vehicles
- Self-Driving Cars: AI algorithms process data from sensors to navigate roads, avoid obstacles, and make driving decisions.
- Drones: AI enables drones to perform tasks such as delivery, surveillance, and mapping autonomously.
- Autonomous Trucks: AI systems help trucks drive long distances, optimize routes, and manage logistics.
- Robotaxis: AI powers ride-hailing services with self-driving cars, providing convenient and efficient transportation.
- Autonomous Buses: AI-driven buses offer public transportation solutions with improved safety and efficiency.
- Agricultural Vehicles: AI helps tractors and harvesters operate autonomously, increasing productivity in farming.
- Mining Vehicles: AI enables mining equipment to operate in hazardous environments without human intervention.
- Marine Vessels: AI systems guide ships and boats, improving navigation and reducing the risk of accidents.
- Autonomous Trains: AI controls trains, optimizing schedules and enhancing safety.
- Delivery Robots: AI-driven robots deliver packages and goods, providing efficient last-mile delivery solutions.
Case Studies
Case Study 1: Tesla’s Autopilot
Challenge: Tesla aimed to create a self-driving car system that could navigate complex urban environments and highways safely.
Solution: Tesla developed the Autopilot system, which uses AI algorithms, cameras, and sensors to process real-time data and make driving decisions.
Result: Tesla’s Autopilot has significantly improved driving safety and convenience, reducing the number of accidents and enhancing the overall driving experience.
Case Study 2: Waymo’s Self-Driving Technology
Challenge: Waymo sought to develop a fully autonomous ride-hailing service that could operate in various weather conditions and traffic scenarios.
Solution: Waymo implemented advanced AI technologies, including machine learning and computer vision, to create a robust self-driving system.
Result: Waymo’s self-driving cars have successfully completed millions of miles on public roads, providing safe and reliable transportation to passengers.
Case Study 3: Uber’s Autonomous Freight
Challenge: Uber aimed to revolutionize the freight industry by introducing autonomous trucks that could transport goods efficiently and safely.
Solution: Uber developed AI-powered autonomous trucks equipped with sensors and cameras to navigate highways and optimize routes.
Result: Uber’s autonomous trucks have demonstrated the potential to reduce transportation costs, improve delivery times, and enhance road safety.
Benefits and Challenges
Benefits:
- Safety: AI reduces human error, leading to fewer accidents.
- Efficiency: Autonomous vehicles optimize routes and reduce fuel consumption.
- Convenience: Self-driving cars offer a hassle-free transportation experience.
Challenges:
- Regulation: Developing and implementing regulations for autonomous vehicles is complex.
- Technology: Ensuring AI systems can handle all driving scenarios remains a challenge.
- Public Acceptance: Gaining public trust in autonomous vehicles is crucial for widespread adoption.
AI in autonomous vehicles is transforming the transportation industry, offering numerous benefits while also presenting challenges that need to be addressed. By understanding these applications and real-world examples, businesses can harness the power of AI to drive innovation and improve operations.
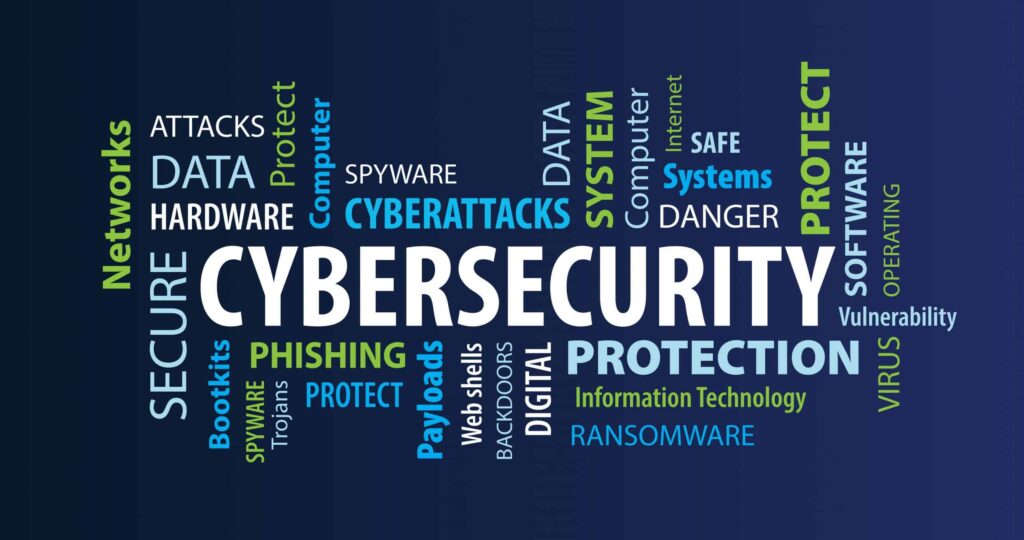
AI Applications for Cybersecurity
Top 10 Applications of AI in Cybersecurity
- Threat Detection: AI algorithms analyze network traffic to identify potential threats and anomalies.
- Incident Response: AI systems automate the response to security incidents, reducing response times and mitigating damage.
- Vulnerability Management: AI identifies and prioritizes vulnerabilities in systems and applications, helping organizations address them proactively.
- Fraud Detection: AI detects fraudulent activities by analyzing patterns and anomalies in transaction data.
- Phishing Detection: AI identifies phishing emails and websites, protecting users from malicious attacks.
- Malware Analysis: AI analyzes malware to understand its behavior and develop effective countermeasures.
- User Behavior Analytics: AI monitors user behavior to detect unusual activities that may indicate a security breach.
- Network Security: AI enhances network security by identifying and blocking malicious traffic.
- Endpoint Protection: AI protects endpoints such as computers and mobile devices from cyber threats.
- Security Information and Event Management (SIEM): AI improves SIEM systems by analyzing security data and providing actionable insights.
Case Studies
Case Study 1: AI-Driven Threat Detection at SecureTech
Challenge: SecureTech faced increasing cyber threats and needed a more effective way to detect and respond to them.
Solution: SecureTech implemented an AI-driven threat detection system that analyzes network traffic and identifies potential threats in real-time.
Result: The AI system improved threat detection accuracy by 40% and reduced response times by 50%.
Case Study 2: AI in Fraud Detection at FinGuard
Challenge: FinGuard needed to detect and prevent fraudulent activities in real-time to protect its customers.
Solution: FinGuard deployed an AI-based fraud detection system that analyzes transaction data and identifies patterns indicative of fraud.
Result: The AI system reduced fraudulent activities by 60% and improved customer trust.
Case Study 3: AI for Phishing Detection at EmailSecure
Challenge: EmailSecure needed to protect its users from phishing attacks, which were becoming increasingly sophisticated.
Solution: EmailSecure implemented an AI-powered phishing detection system that analyzes emails and identifies phishing attempts.
Result: The AI system reduced phishing attacks by 70% and improved user security.
Benefits and Challenges
Benefits:
- Enhanced Security: AI improves threat detection and response, reducing the risk of cyber attacks.
- Efficiency: AI automates security tasks, freeing up human resources for more complex activities.
- Proactive Defense: AI identifies and addresses vulnerabilities before they can be exploited.
Challenges:
- Data Privacy: Ensuring the privacy and security of data used by AI systems is crucial.
- Bias: AI systems can be biased if trained on incomplete or unrepresentative data.
- Implementation Costs: The initial investment in AI technology can be high.
AI in cybersecurity is transforming the way organizations protect their systems and data. By understanding these applications and real-world examples, businesses can harness the power of AI to enhance their security posture and mitigate cyber threats.
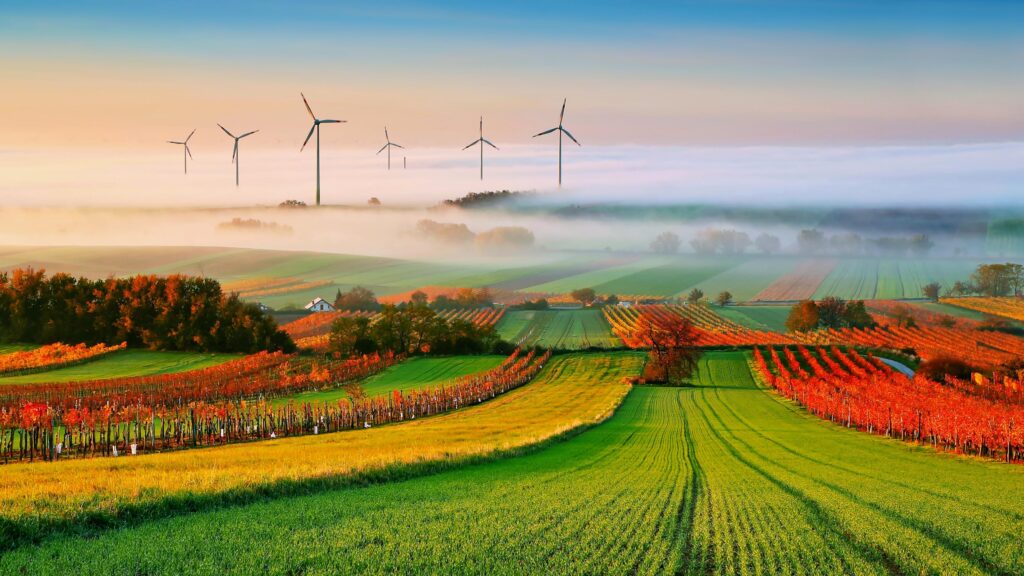
AI Applications for Agriculture
Top 10 Applications of AI in Agriculture
- Precision Farming: AI analyzes data from sensors and satellites to optimize crop yields and resource usage.
- Crop Monitoring: AI systems monitor crop health using drones and satellite imagery, identifying issues early.
- Livestock Management: AI tracks animal health and behavior, improving livestock care and productivity.
- Irrigation Management: AI optimizes water usage by analyzing soil moisture and weather data.
- Pest and Disease Detection: AI identifies pests and diseases in crops, enabling timely interventions.
- Soil Health Monitoring: AI analyzes soil samples to assess nutrient levels and recommend treatments.
- Yield Prediction: AI predicts crop yields based on historical data and current conditions.
- Automated Harvesting: AI-powered robots harvest crops efficiently, reducing labor costs.
- Supply Chain Optimization: AI optimizes the agricultural supply chain, from production to distribution.
- Market Forecasting: AI predicts market trends and prices, helping farmers make informed decisions.
Case Studies
Case Study 1: Precision Farming at SmartAg
Challenge: SmartAg needed to optimize crop yields and reduce resource usage.
Solution: SmartAg implemented an AI system that analyzes data from sensors and satellites to provide real-time recommendations for planting, fertilizing, and irrigating crops.
Result: The AI system increased crop yields by 20% and reduced water usage by 15%.
Case Study 2: Crop Monitoring at AgroTech
Challenge: AgroTech faced challenges in monitoring crop health across large fields.
Solution: AgroTech deployed AI-powered drones that capture high-resolution images of crops. The AI system analyzes these images to identify signs of pests, diseases, and nutrient deficiencies.
Result: The AI-powered crop monitoring system reduced crop losses by 25% and improved overall crop health.
Case Study 3: Livestock Management at FarmSense
Challenge: FarmSense needed to improve livestock health and productivity.
Solution: FarmSense implemented an AI system that tracks animal health and behavior using sensors and cameras. The AI system provides insights into feeding, breeding, and health management.
Result: The AI system improved livestock productivity by 30% and reduced health-related issues by 20%.
Benefits and Challenges
Benefits:
- Increased Efficiency: AI optimizes farming practices, leading to higher efficiency and productivity.
- Resource Management: AI helps manage resources like water and fertilizers more effectively.
- Improved Crop Health: AI systems monitor and improve crop health, reducing losses and increasing yields.
Challenges:
- Implementation Costs: The initial investment in AI technology can be high.
- Data Privacy: Ensuring the privacy and security of data used by AI systems is crucial.
- Skill Gap: There is a need for skilled personnel to develop, implement, and maintain AI applications.
AI in agriculture is transforming the farming industry, offering numerous benefits while also presenting challenges that need to be addressed. By understanding these applications and real-world examples, businesses can harness the power of AI to drive innovation and improve operations.
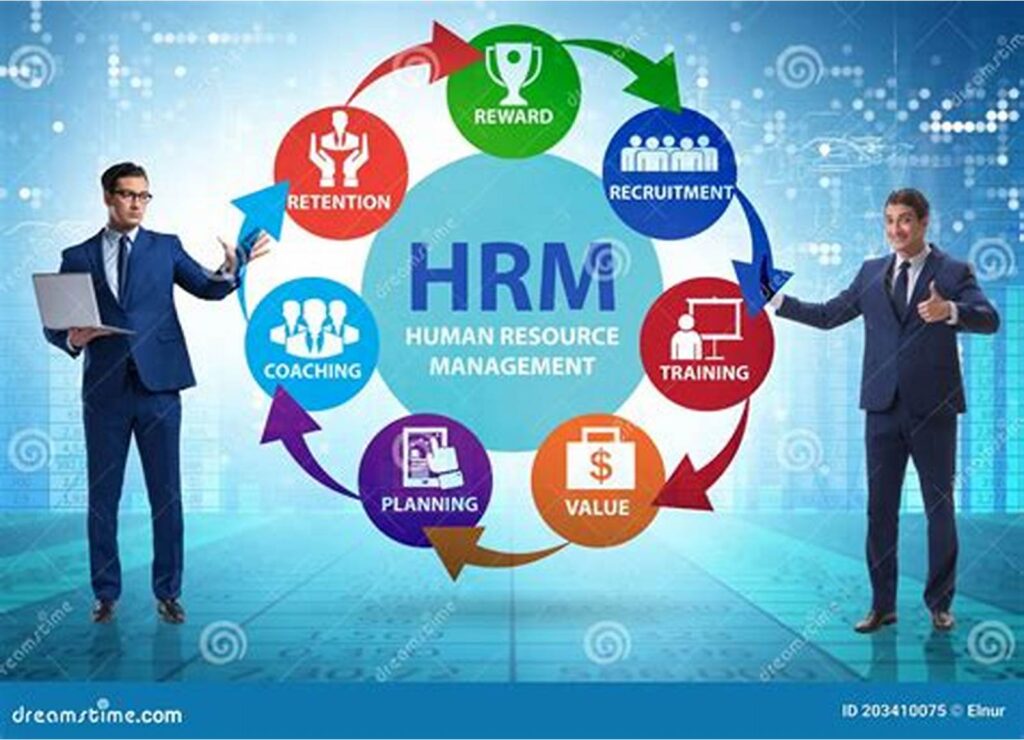
AI Applications for Human Resources
Top 10 Applications of AI in Human Resources
- Talent Acquisition: AI helps identify and attract top talent by analyzing resumes and matching candidates to job openings.
- Employee Engagement: AI systems monitor employee sentiment and engagement through surveys and feedback analysis.
- Performance Management: AI evaluates employee performance and provides insights for improvement.
- Onboarding: AI-powered chatbots guide new hires through the onboarding process, answering questions and providing information.
- Training and Development: AI recommends personalized training programs based on employee skills and career goals.
- Workforce Planning: AI predicts future workforce needs and helps plan for hiring and training.
- Employee Retention: AI identifies factors that contribute to employee turnover and suggests strategies to improve retention.
- Compensation Management: AI analyzes market data to recommend competitive salaries and benefits.
- Diversity and Inclusion: AI helps identify and address biases in hiring and promotion processes.
- HR Analytics: AI provides insights into HR metrics, helping organizations make data-driven decisions.
Case Studies
Case Study 1: AI-Driven Talent Acquisition at TechHire
Challenge: TechHire struggled to find qualified candidates for specialized tech roles.
Solution: TechHire implemented an AI-powered talent acquisition system that analyzes resumes and matches candidates to job openings based on skills and experience.
Result: The AI system reduced the time to fill positions by 30% and improved the quality of hires.
Case Study 2: Employee Engagement at HappyCorp
Challenge: HappyCorp needed to improve employee engagement and reduce turnover.
Solution: HappyCorp deployed an AI system that monitors employee sentiment through surveys and feedback analysis. The system provides insights into employee engagement and suggests strategies for improvement.
Result: The AI system increased employee engagement by 25% and reduced turnover by 15%.
Case Study 3: Performance Management at PerformWell
Challenge: PerformWell faced challenges in evaluating employee performance and providing actionable feedback.
Solution: PerformWell implemented an AI-driven performance management system that evaluates employee performance based on various metrics and provides personalized feedback.
Result: The AI system improved performance evaluation accuracy by 20% and increased employee satisfaction with feedback.
Benefits and Challenges
Benefits:
- Efficiency: AI automates routine HR tasks, freeing up HR professionals to focus on strategic activities.
- Data-Driven Decisions: AI provides insights based on data, helping organizations make informed HR decisions.
- Improved Employee Experience: AI enhances various HR processes, leading to a better employee experience.
Challenges:
- Data Privacy: Ensuring the privacy and security of employee data is crucial.
- Bias: AI systems can be biased if trained on incomplete or unrepresentative data.
- Implementation Costs: The initial investment in AI technology can be high.
AI in Human Resources is transforming the way organizations manage their workforce. By understanding these applications and real-world examples, businesses can harness the power of AI to improve HR processes and enhance employee satisfaction.
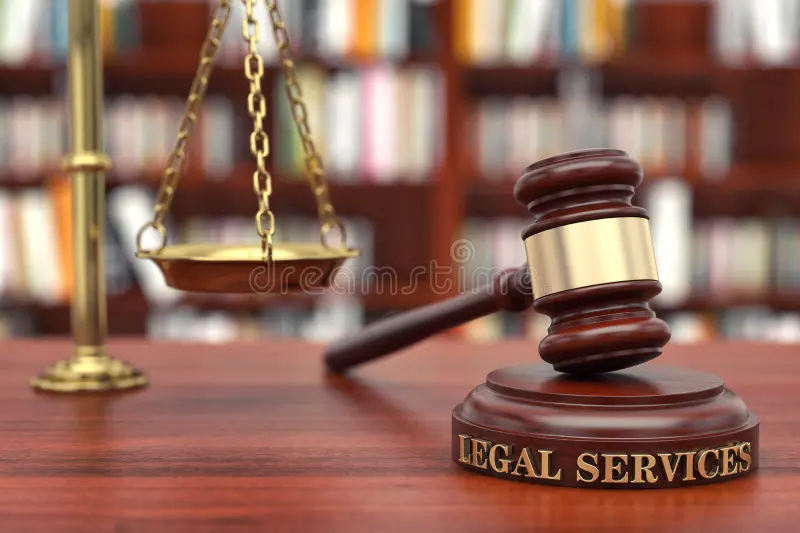
AI Applications for Legal Services
Top 10 Applications of AI in Legal Services
- Legal Research: AI systems analyze vast amounts of legal documents to find relevant information quickly.
- Contract Analysis: AI reviews and analyzes contracts to identify key terms, potential risks, and compliance issues.
- Case Prediction: AI predicts the outcomes of legal cases based on historical data and case law.
- Document Automation: AI automates the creation of legal documents, reducing time and errors.
- E-Discovery: AI assists in the discovery process by identifying relevant documents and information in large datasets.
- Due Diligence: AI conducts due diligence by analyzing financial records, contracts, and other documents.
- Litigation Support: AI provides support during litigation by organizing and analyzing case information.
- Compliance Monitoring: AI monitors compliance with regulations and identifies potential violations.
- Intellectual Property Management: AI helps manage intellectual property by analyzing patents, trademarks, and copyrights.
- Client Management: AI enhances client management by analyzing client data and providing insights for better service.
Case Studies
Case Study 1: AI-Driven Legal Research at LexisNexis
Challenge: LexisNexis needed to improve the efficiency and accuracy of legal research for its users.
Solution: LexisNexis implemented an AI-powered legal research system that analyzes vast amounts of legal documents and provides relevant information quickly.
Result: The AI system reduced the time required for legal research by 50% and improved the accuracy of search results.
Case Study 2: Contract Analysis at JPMorgan Chase
Challenge: JPMorgan Chase faced challenges in reviewing and analyzing a large volume of contracts efficiently.
Solution: JPMorgan Chase deployed an AI-based contract analysis system that reviews contracts, identifies key terms, and highlights potential risks.
Result: The AI system reduced the time required for contract analysis by 80% and improved risk identification.
Case Study 3: Case Prediction at Blue J Legal
Challenge: Blue J Legal aimed to predict the outcomes of legal cases to assist lawyers in making informed decisions.
Solution: Blue J Legal developed an AI system that analyzes historical case data and predicts the outcomes of legal cases.
Result: The AI system achieved an accuracy rate of 90% in predicting case outcomes, helping lawyers make better-informed decisions.
Benefits and Challenges
Benefits:
- Efficiency: AI automates routine legal tasks, freeing up lawyers to focus on more complex activities.
- Accuracy: AI improves the accuracy of legal research, contract analysis, and case predictions.
- Cost Reduction: AI reduces the time and costs associated with legal processes.
Challenges:
- Data Privacy: Ensuring the privacy and security of legal data is crucial.
- Bias: AI systems can be biased if trained on incomplete or unrepresentative data.
- Implementation Costs: The initial investment in AI technology can be high.
AI in legal services is transforming the industry by enhancing efficiency, accuracy, and cost-effectiveness. By understanding these applications and real-world examples, businesses can harness the power of AI to improve their legal processes and services.
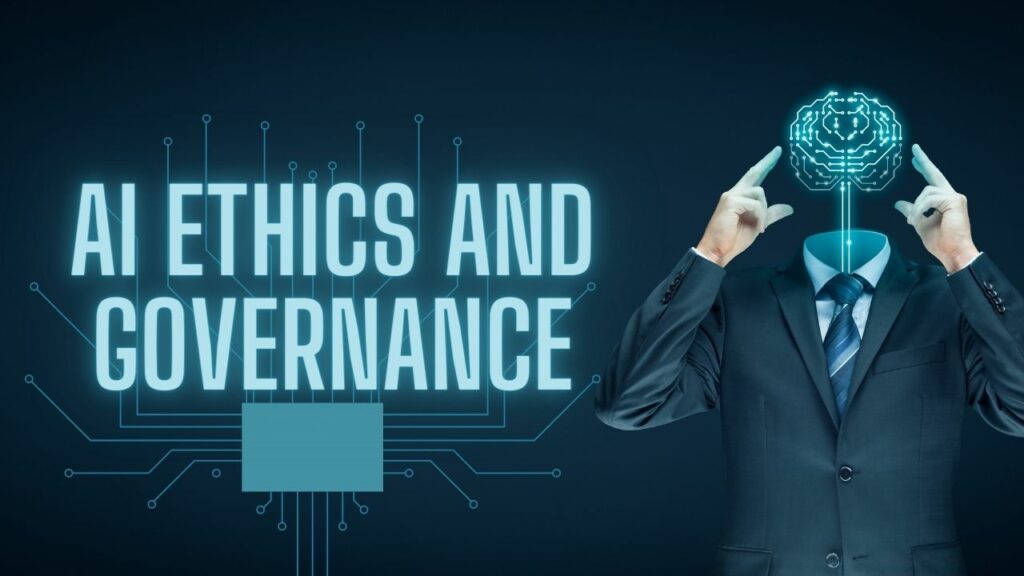
AI Ethics and Governance
Top 10 Ethical Considerations in AI
- Data Privacy: Ensuring the privacy and security of data used by AI systems.
- Bias and Fairness: Addressing biases in AI algorithms to ensure fair treatment for all users.
- Transparency: Making AI systems and their decision-making processes understandable to users.
- Accountability: Holding developers and organizations accountable for the actions of their AI systems.
- Safety: Ensuring that AI systems operate safely and do not cause harm.
- Human Oversight: Maintaining human control over AI systems to prevent unintended consequences.
- Informed Consent: Ensuring that users are aware of and consent to the use of AI systems.
- Ethical Use of Data: Using data ethically and respecting the rights of data subjects.
- Environmental Impact: Considering the environmental impact of AI technologies and promoting sustainability.
- Social Impact: Assessing the broader social implications of AI and ensuring that it benefits society as a whole.
Case Studies
Case Study 1: Data Privacy at Apple
Challenge: Apple needed to ensure the privacy and security of user data while using AI to enhance its products and services.
Solution: Apple implemented strong data encryption and anonymization techniques. The company also introduced features like “Sign in with Apple” to minimize data collection.
Result: Apple successfully enhanced user privacy and security, gaining customer trust and setting a high standard for data privacy in the tech industry.
Case Study 2: Bias and Fairness at IBM
Challenge: IBM faced challenges in ensuring that its AI systems were free from biases that could lead to unfair treatment of users.
Solution: IBM developed the AI Fairness 360 toolkit, an open-source library that helps developers detect and mitigate biases in AI models.
Result: The toolkit improved the fairness of IBM’s AI systems and provided a valuable resource for the broader AI community.
Case Study 3: Transparency at Google
Challenge: Google needed to make its AI systems more transparent and understandable to users.
Solution: Google introduced the “Explainable AI” initiative, which focuses on developing AI models that can provide clear explanations for their decisions.
Result: The initiative improved the transparency of Google’s AI systems, helping users understand how decisions are made and increasing trust in AI technologies.
Benefits and Challenges
Benefits:
- Trust: Ethical AI practices build trust with users and stakeholders.
- Fairness: Addressing biases ensures fair treatment for all users.
- Accountability: Clear accountability mechanisms hold developers and organizations responsible for their AI systems.
Challenges:
- Complexity: Implementing ethical AI practices can be complex and resource-intensive.
- Regulation: Navigating the regulatory landscape for AI can be challenging.
- Evolving Standards: Ethical standards for AI are constantly evolving, requiring ongoing adaptation.
AI ethics and governance are crucial for ensuring that AI technologies are developed and deployed responsibly. By understanding these ethical considerations and real-world examples, businesses can harness the power of AI while upholding ethical standards and promoting trust.
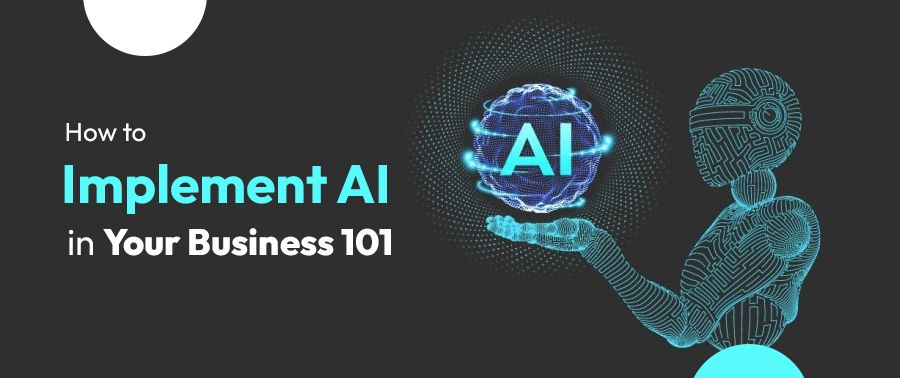
Implementing AI in Your Business
Top 10 Applications of AI in Business
- Customer Service: AI-powered chatbots provide 24/7 support, answering queries and resolving issues quickly.
- Sales and Marketing: AI analyzes customer data to create personalized marketing campaigns and improve sales strategies.
- Supply Chain Management: AI optimizes supply chain processes, predicting demand and managing inventory efficiently.
- Human Resources: AI streamlines recruitment, employee engagement, and performance management.
- Finance: AI detects fraud, assesses credit risk, and automates financial processes.
- Manufacturing: AI enhances predictive maintenance, quality control, and automation.
- Healthcare: AI improves diagnostics, treatment planning, and patient care.
- Retail: AI personalizes marketing, manages inventory, and enhances customer service.
- Energy Management: AI optimizes energy consumption and predicts maintenance needs.
- Legal Services: AI assists in legal research, contract analysis, and case prediction.
Creating an AI Implementation Strategy
Defining an AI implementation strategy involves several key steps to ensure that AI technologies are effectively integrated into your business processes. Here’s a comprehensive guide to help you get started:
Identifying AI Opportunities
- Conduct AI Assessments: Begin by evaluating your current business processes to identify areas where AI can provide the most significant impact. Look for repetitive tasks, data-intensive processes, and areas with high error rates.
- Analyze Business Processes: Map out your business processes and identify pain points that AI can address. Consider how AI can improve efficiency, reduce costs, and enhance customer experiences.
Building an AI Strategy
- Set Clear AI-Related Goals and Objectives: Define what you want to achieve with AI. This could include improving customer service, increasing operational efficiency, or enhancing decision-making processes.
- Develop a Roadmap for AI Implementation: Create a detailed plan outlining the steps needed to implement AI in your business. This should include timelines, milestones, and key performance indicators (KPIs) to measure success.
- Build an Internal AI Team or Partner with External AI Providers: Decide whether to develop an in-house AI team or collaborate with external AI providers. An internal team offers more control, while external providers can bring specialized expertise and resources.
Addressing Ethical Considerations
Transparency and Explainability of AI Decisions: Make sure that AI decisions are transparent and explainable. This helps build trust with stakeholders and ensures accountability.
Data Privacy and Security: Ensure that your AI systems comply with data privacy regulations and implement robust security measures to protect sensitive information.
Bias and Fairness in AI Algorithms: Address potential biases in your AI algorithms by using diverse and representative data sets. Regularly audit your AI systems to ensure fairness and accuracy.
Case Studies
Case Study 1: AI in Customer Service at TechSupport Inc.
Challenge: TechSupport Inc. faced long wait times and high customer dissatisfaction due to limited customer service resources.
Solution: TechSupport Inc. implemented an AI-powered chatbot that provides 24/7 customer support. The chatbot handles common queries and resolves issues quickly.
Result: The AI chatbot reduced customer wait times by 60% and improved customer satisfaction by 40%.
Case Study 2: AI in Sales and Marketing at MarketGenius
Challenge: MarketGenius struggled to engage customers with generic marketing campaigns and needed a way to personalize their efforts.
Solution: MarketGenius deployed an AI system that analyzes customer data to create personalized marketing campaigns tailored to individual preferences.
Result: The personalized marketing campaigns led to a 35% increase in sales and a 25% improvement in customer engagement.
Case Study 3: AI in Supply Chain Management at LogisticsPro
Challenge: LogisticsPro faced challenges in managing inventory levels and predicting demand accurately.
Solution: LogisticsPro adopted an AI system that analyzes historical sales data and external factors to predict demand and optimize inventory management.
Result: The AI-driven supply chain management system reduced stockouts by 30% and decreased excess inventory by 20%.
The Future of AI in Business
Emerging trends in AI, such as edge computing, AIoT (AI of Things), and explainable AI, are shaping the future of business. These technologies will further enhance AI’s capabilities and impact on various industries.
Implementing AI in your business can revolutionize operations, improve efficiency, and drive innovation. By understanding these applications and real-world examples, businesses can harness the power of AI to achieve their goals and stay competitive in the ever-evolving market.
Conclusion
Throughout this book, we’ve explored the transformative power of AI across various industries. From healthcare to finance, retail to manufacturing, and beyond, AI is revolutionizing the way businesses operate. We’ve seen how AI enhances diagnostics, improves customer service, optimizes supply chains, and personalizes marketing efforts. Each chapter provided real-world case studies, showcasing the tangible benefits and challenges of AI implementation.
In healthcare, AI improves diagnostics, treatment planning, and patient care. Finance benefits from AI in fraud detection, risk management, and personalized services. Retail and e-commerce leverage AI for personalized marketing, inventory management, and customer service. Manufacturing sees enhancements in predictive maintenance, quality control, and automation. Telecommunications uses AI for network optimization and customer service. Education benefits from personalized learning and administrative automation. Energy and utilities optimize consumption and grid management with AI. Media and entertainment utilize AI for content recommendation and production automation. Government and public sector employ AI for public services and resource allocation. Autonomous vehicles rely on AI for self-driving technology. Cybersecurity uses AI for threat detection and incident response. Agriculture benefits from precision farming and crop monitoring. Human resources streamline recruitment and performance management with AI. Legal services enhance research and contract analysis with AI. Finally, we discussed the importance of AI ethics and governance, addressing data privacy, bias, and transparency.
Future of AI
The future of AI holds exciting possibilities. Emerging trends such as edge computing, AIoT (AI of Things), and explainable AI will further enhance AI’s capabilities. Edge computing will bring AI processing closer to data sources, reducing latency and improving efficiency. AIoT will integrate AI with the Internet of Things, creating smarter and more connected devices. Explainable AI will make AI systems more transparent and understandable, fostering trust and accountability.
AI will continue to impact the future of work, automating routine tasks and enabling employees to focus on more complex and creative activities. Businesses that embrace AI will gain a competitive edge, driving innovation and improving operations.
Call to Action
As we conclude this journey into the world of AI, I encourage you to explore AI applications in your own business and industry. Identify areas where AI can provide the most significant impact and develop a strategy for implementation. Embrace the ethical considerations and ensure that your AI systems are fair, transparent, and accountable. Stay informed about emerging trends and continuously adapt to the evolving AI landscape.
The potential of AI is vast, and by harnessing its power, you can revolutionize your business and stay ahead in today’s competitive market. Let’s embark on this exciting journey together and unlock the full potential of AI for a brighter future.
Appendices
Glossary of AI Terms
A
Algorithm: A set of step-by-step instructions for solving a problem or performing a task. In AI, algorithms help machines learn from data and make decisions.
Artificial Intelligence (AI): The simulation of human intelligence in machines that are programmed to think and learn like humans.
B
Bias: A systematic error in AI systems that can lead to unfair or inaccurate outcomes. Bias can occur if the training data is not representative of the real world.
Big Data: Large and complex datasets that require advanced tools and techniques to analyze and extract valuable information.
C
Chatbot: An AI-powered program that can simulate a conversation with users, typically used for customer service and support.
Computer Vision: A field of AI that enables machines to interpret and understand visual information from the world, such as images and videos.
Convolutional Neural Network (CNN): A type of deep learning algorithm commonly used for image recognition and processing.
D
Data Mining: The process of discovering patterns and insights from large datasets using statistical and computational techniques.
Deep Learning: A subset of machine learning that uses neural networks with many layers to learn from large amounts of data.
E
Edge Computing: A computing paradigm that brings data processing closer to the data source, reducing latency and improving efficiency.
Explainable AI (XAI): AI systems designed to provide clear and understandable explanations for their decisions and actions.
F
Feature Extraction: The process of identifying and selecting relevant features from raw data to improve the performance of machine learning models.
Fraud Detection: The use of AI to identify and prevent fraudulent activities by analyzing patterns and anomalies in data.
G
Generative Adversarial Network (GAN): A type of neural network used to generate new data samples that are similar to a given dataset.
Gradient Descent: An optimization algorithm used to minimize the error in machine learning models by adjusting the model’s parameters.
H
Hyperparameter: A parameter that is set before the training process of a machine learning model and affects its performance.
Human-in-the-Loop (HITL): A model of AI development where human input and feedback are integrated into the AI system to improve its performance.
I
Internet of Things (IoT): A network of interconnected devices that collect and exchange data, often enhanced by AI for smarter decision-making.
Intelligent Agent: An autonomous entity that observes its environment and takes actions to achieve specific goals.
J
Joint Probability: The probability of two events occurring simultaneously, used in probabilistic models and AI algorithms.
K
Knowledge Base: A repository of structured information that an AI system uses to make decisions and provide answers.
K-Nearest Neighbors (KNN): A simple machine learning algorithm used for classification and regression tasks.
L
Linear Regression: A statistical method used to model the relationship between a dependent variable and one or more independent variables.
Logistic Regression: A machine learning algorithm used for binary classification tasks.
M
Machine Learning (ML): A subset of AI that enables machines to learn from data and improve their performance over time without being explicitly programmed.
Natural Language Processing (NLP): A field of AI that enables machines to understand, interpret, and respond to human language.
N
Neural Network: A computational model inspired by the human brain, consisting of interconnected nodes (neurons) that process information.
Normalization: The process of scaling data to a standard range to improve the performance of machine learning models.
O
Overfitting: A situation where a machine learning model performs well on training data but poorly on new, unseen data due to excessive complexity.
Optimization: The process of finding the best solution or parameters for a machine learning model to minimize error and improve performance.
P
Predictive Analytics: The use of AI and statistical techniques to analyze historical data and make predictions about future events.
Principal Component Analysis (PCA): A dimensionality reduction technique used to reduce the number of features in a dataset while preserving important information.
Q
Quantum Computing: An emerging field of computing that uses quantum mechanics to perform complex calculations much faster than classical computers.
Query: A request for information or data from a database or AI system.
R
Reinforcement Learning (RL): A type of machine learning where an agent learns to make decisions by receiving rewards or penalties for its actions.
Robotics: The field of engineering and AI that involves the design, construction, and operation of robots.
S
Supervised Learning: A type of machine learning where the model is trained on labeled data, learning to map inputs to outputs.
Support Vector Machine (SVM): A machine learning algorithm used for classification and regression tasks.
T
Training Data: The dataset used to train a machine learning model, containing input-output pairs for supervised learning.
Transfer Learning: A technique where a pre-trained model is adapted to a new, but related, task.
U
Unsupervised Learning: A type of machine learning where the model is trained on unlabeled data, learning to identify patterns and relationships.
Underfitting: A situation where a machine learning model performs poorly on both training and new data due to insufficient complexity.
V
Validation Data: A dataset used to evaluate the performance of a machine learning model during training, helping to tune hyperparameters.
Virtual Assistant: An AI-powered program that can perform tasks or services for an individual based on commands or questions.
W
Weight: A parameter in a neural network that is adjusted during training to minimize error and improve performance.
Word Embedding: A representation of words in a continuous vector space, used in natural language processing to capture semantic relationships.
X
XGBoost: An optimized gradient boosting algorithm used for supervised learning tasks, known for its high performance and efficiency.
Y
Yield Prediction: The use of AI to predict crop yields based on historical data and current conditions, commonly used in agriculture.
Z
Zero-Shot Learning: A machine learning technique where the model can recognize and classify new, unseen classes without having been explicitly trained on them.
This glossary provides a comprehensive overview of key AI terms and concepts, helping you navigate the world of artificial intelligence with ease.
Resources for Further Reading on AI
Books
- The Worlds I See: Curiosity, Exploration, and Discovery at the Dawn of AI by Fei-Fei Li (2023)
- An engaging memoir by a trailblazing computer scientist, providing an insider’s view on AI advancements and potential risks.
- The Alignment Problem by Brian Christian (2020)
- Explores the ethical and technical challenges of aligning AI systems with human values.
- Artificial Intelligence: A Guide for Thinking Humans by Melanie Mitchell (2019)
- Offers a human-centered perspective on AI, discussing its history, capabilities, and challenges.
- Genius Makers by Cade Metz (2021)
- Chronicles the pioneers of AI and their groundbreaking work.
- AI Superpowers: China, Silicon Valley, and the New World Order by Kai-Fu Lee (2018)
- Examines the global AI race and its implications for the future.
Articles
- AI Prompt Engineering Is Dead by Dina Genkina (2024)
- Discusses the decline of prompt engineering as AI models become better at constructing prompts themselves.
- Generative AI Has a Visual Plagiarism Problem by Gary Marcus and Reid Southen (2024)
- Investigates the issue of visual plagiarism in generative AI systems.
- Five Trends in AI and Data Science for 2025 by Thomas H. Davenport and Randy Bean (2025)
- Highlights emerging AI trends that leaders should monitor.
- Elon Musk agrees that we’ve exhausted AI training data by Kyle Wiggers (2025)
- Explores the challenges of finding new training data for AI models and the shift towards synthetic data.
- OpenAI Shuts Down Developer Who Made AI-Powered Gun Turret by Gizmodo (2025)
- Covers the ethical and safety concerns surrounding the use of AI in weaponry.
Websites
- MIT News – Artificial Intelligence: Stay updated with the latest AI research and developments from MIT.
- IEEE Spectrum – AI Analysis: Features in-depth articles and analysis on AI technologies and their impact.
- DigitalOcean – AI Books: A curated list of essential AI books that cover fundamental concepts, applications, and future trends.
- Guides.ai – Best AI Websites: A guide to the top AI websites and apps, including tools for chatbots, image generation, and more.
- Aixploria – Free AI Tools: A comprehensive list of free AI tools available online, categorized by functionality.
These resources provide a wealth of information for anyone looking to deepen their understanding of AI and stay informed about the latest developments in the field.